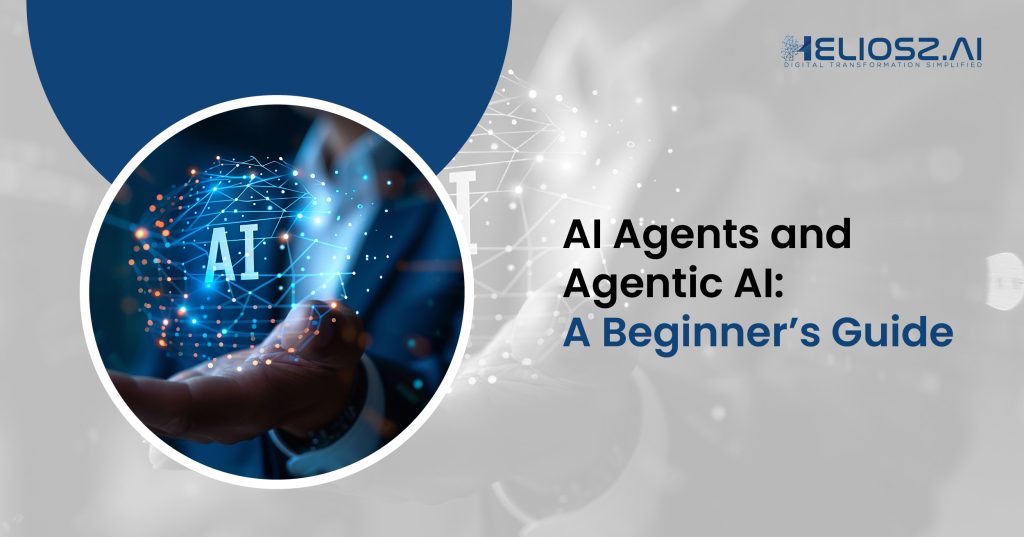
Artificial Intelligence (AI) keeps improving. It’s not just a matter of following a simple command anymore, but it’s becoming more autonomous and doing things by itself. Picture machines are not only able to comprehend information but also decide what comes next and act accordingly. That is the direction the concept of AI agents and Agentic AI take. They are a giant leap forward, making machines more akin to intelligent assistants that can reason and act independently.
Consider it: rather than constantly requiring someone to instruct a computer on what to do every step of the way, these new types of AI are able to observe a situation, determine what should be done, and then do it. This is occurring in a lot of areas of technology, and getting a handle on what AI agents and Agentic AI are all about is becoming super important for just about anyone. Whether you’re simply interested in new technology or you use computers all the time, learning about these intelligent, standalone machines will enable you to make sense of the future.
This informative article is here to define AI agents and Agentic AI simply and without getting overly complicated. We’ll see what they are, how they work, and where you can find them in the real world. By the end, you’ll have a clear idea of what these clever, acting machines are all about and why they’re important.
What is an AI Agent?
By its definition, an AI agent is a computational agent, which is most commonly realized in the form of a software program or an advanced integrated system, functioning in a specific environment and demonstrating a circular process of perception, processing, decision-making, and action. An AI agent’s main mission is to independently work towards achieving designated goals through intelligent interaction with its environment.
Key Features of AI Agents
Environmental Perception through Sensors or Inputs: An AI agent has the ability to receive information regarding its functioning environment through diverse sensory modalities or input processes. These include physical sensors within robotic systems and networked environmental data streams up to natural language processing modules reading linguistic input and computer vision modules processing visual input. The perception subsystem is in charge of converting raw environmental stimulation into a processable internal representation.
a) Information Processing and Inferential Reasoning
When it perceives its surroundings, the AI agent goes through the pivotal process of processing and interpreting the data it has gathered. This is done by utilizing an internal knowledge representation, potentially in the form of pre-installed rules, statistical models that have been developed through machine learning, or symbolic knowledge structures. The agent uses inferential reasoning processes to infer higher-level conclusions, comprehend the present situation of the environment in terms of its objectives, and anticipate the possible effects of different actions.
b) Autonomous Decision Making
From the information processed and the results of its reasoning activities, the AI agent can make decisions about the best course of action. This decision-making process consists of considering various available options, determining their likely effect on achieving goals, and choosing the action that optimizes a given utility function or is most in line with the agent’s goals. The degree of autonomy suggests that the agent is able to make these decisions without needing explicit, sequential human control.
c) Independent Action Execution
As a sequel to the decision-making stage, the AI agent has the capability to perform actions in its world. The character of those actions depends on the embodiment and domain of the agent, varying from producing linguistic responses and data manipulation in computer systems to driving physical actuators in robot systems or calling functions in external software interfaces.
d) Goal-Directed Operational Framework
The overall operational framework of an AI agent is goal-directed in nature. All operations, ranging from environmental perception to action selection, are ultimately directed towards the accomplishment of one or more designated goals. The goal supplies the performance criteria for the agent’s functioning and directs its strategic decision-making.
What is an Agentic AI?
Beyond the traditional approach of using single, standalone AI agents, Agentic AI represents a broader architectural paradigm that often involves multiple interacting agents, commonly referred to as Multi-Agent Systems (MAS). Instead of relying on the capabilities of a single AI system, Agentic AI leverages the principles of distributed intelligence—where different agents contribute specialized skills, operate in parallel, and adapt to changing situations. Each agent typically has its own goals, knowledge base, and ability to act, but they are designed to coexist and collaborate within a shared environment to achieve broader, system-level objectives. While Agentic AI is frequently implemented through multi-agent coordination, it also emphasizes the design of AI systems with high levels of autonomy, proactiveness, and self-direction—even when composed of just one sophisticated agent.
Key Characteristics of Agentic AI Systems
a) Task Decomposition to Parallel Processing
Complex problems are typically broken down into small, manageable sub-problems, which can be assigned to respective agents based on their specialized skill and knowledge. This enables parallel processing and efficient utilization of computational resources.
b) System Scalability and Modularity for Adaptation
The modularity of the Agentic AI architecture, such that the system is composed of independent agents, enables scalability as well as adaptation. New agents with different capacities can be simply integrated into the system, while the already-existing agents can be updated or revised without a full system redesign.
c) Improved System Fault Tolerance and Robustness
The distributed nature of the Agentic AI systems also leads to improved fault tolerance and robustness. In case some of the agents fail, other agents can still work and possibly replace the lost abilities, leading to a more resilient system.
d) Inter-Agent Negotiation and Collaboration Mechanisms
Maybe the most characteristic feature of Agentic AI is the existence of mechanisms through which agents negotiate, exchange information, coordinate their actions, and even participate in negotiation processes to resolve disagreements and optimize the system’s overall performance. These mechanisms range from simple message passing protocols to game-theory-based negotiation or auction-based mechanisms.
Agentic AI vs AI Agents: Key Differences
Feature | AI Agents | Agentic AI |
Scope | Individual intelligent systems for specific tasks. | Broader paradigm of highly autonomous systems for complex goals. |
Autonomy Level | Can range from limited to moderate; may need more human guidance. | High autonomy; independent decision-making and action. |
Goal Orientation | Focused on specific, often short-term tasks. | Focused on complex, longer-term goals. |
Reasoning & Planning | Basic reasoning and planning within a narrow domain. | Advanced reasoning, contextual analysis, and iterative planning. |
Learning & Adaptation | Learns within a domain; may need retraining for new scenarios. | Dynamic learning and adaptation across contexts. |
Complexity | Can be simple to complex; often for well-defined problems. | Generally more complex, integrating diverse AI technologies. |
Interaction & Collaboration | Primarily interacts with users or systems; less inherent collaboration. | Often involves multiple agents that communicate and collaborate. |
Proactiveness | Can be reactive or somewhat proactive within scope. | More inherently proactive and anticipatory. |
Human Oversight | May require more frequent human intervention. | Designed for less direct human supervision. |
Enterprise-Focused AI Agent Development: Book Your 1:1 Session Today!
Real-world Applications of AI Agents
a) Intelligent Virtual Assistants (IVAs)
Very sophisticated conversational agents that can process natural language, keep context, and perform actions such as scheduling, knowledge retrieval, and device operation.
b) Advanced Conversational AI Platforms
Platforms for more sophisticated and subtle interactions with users, occasionally even including reasoning and planning capabilities in order to be capable of offering more comprehensive assistance.
c) Personalized Recommendation Systems
Computer systems that study users’ habits and interests to provide individualized recommendations for products, services, or content.
d) Autonomous Robotic Platforms
Robots with advanced perception, planning, and control systems that enable them to navigate complex environments and perform tasks with minimal human intervention.
e) Intelligent Process Automation (IPA) Systems
Software agents that automate complex and repetitive business processes by simulating human actions and integrating with multiple enterprise systems.
Example Applications of Agentic AI
a) Swarm Robotic Systems for Distributed Tasks
Thousands of distributed autonomous robots communicating through local interactions to accomplish high-level collective behavior like search and rescue, environment monitoring, or infrastructure inspection.
b) Decentralized Autonomous Organizations (DAOs)
Organizations based on blockchain that are controlled by smart contracts (that are autonomous agents) that determine and allocate resources based on predetermined rules.
c) Multi-Agent Simulation Frameworks
Computational models that utilize interacting AI agents for simulating and analyzing the behavior of complex systems in fields like traffic flow, social processes, and economic markets.
d) AI for Scientific Discovery
Systems where different AI agents, each having a different specific area of expertise in a science problem (e.g., data analysis, hypothesis generation, experimental design), collaborate with each other to speed up the research process.
e) Smart Cyber Defense Architectures
Networks of distributed AI agents that collaborate to monitor network traffic, detect anomalies, analyze threats, and orchestrate automated countermeasures to cyberattacks.
Frequently Asked Questions (FAQs)
What is an AI agent?
An AI agent is a computer system created to sense its surroundings with the help of numerous sensors or inputs, process such information through internal mechanisms of knowledge and reasoning, independently make decisions based on given goals, and take actions in the environment to accomplish those goals. Its primary task is to function intelligently and independently in a particular area with the goal to accomplish its assigned objectives.
Is ChatGPT an AI agent?
Yes, ChatGPT can be identified as a high-level AI agent that acts in the realm of natural language. It receives input as text, processes it through its large language model to determine context and intent, decides on the proper linguistic response, and performs by producing text to accomplish the user’s communicative purpose.
What is agentic in AI?
“Agentic” in AI describes the property of an AI system having the attributes of an agent, i.e., autonomy, goal-oriented behavior, perception of the environment, reasoning, and the capacity to make actions to change its environment to achieve its goals, acting with a level of autonomy independent of direct external input.
What is the difference between generative AI and agentic AI?
Generative AI is centered on generating new data instances that are similar to the training data, like text or images. Agentic AI is centered on generating independent systems that can sense, reason, decide, and act in an environment to reach certain goals. Although an agentic AI system may use generative AI as one of its action modalities, its essential difference is based on its independent and goal-oriented behavior, not merely on data generation.
What is the difference between conversational AI and agentic AI?
Conversational AI is a type of AI focused on facilitating natural language interaction between human and machine. Agentic AI is a wider paradigm covering any AI system showing autonomous, goal-directed behavior. Although a conversational AI system may be agentic if it actively initiates actions to facilitate user goals above and beyond the mere conversation, not all conversational AI have this autonomous, action-based feature.
Conclusion
In summary, AI agents and the architectural model of Agentic AI are a major milestone in the development of artificial intelligence, heading towards the development of more intelligent, autonomous, and cooperative systems. Their ability to perceive, reason, decide, and act autonomously to accomplish specified goals makes them key technologies for solving increasingly complex problems in various industries.
The revolutionary potential of these independent systems ranges across a broad spectrum of uses, from improving human-machine interaction to transforming industrial processes and propelling scientific discovery. With continuing acceleration in AI development, an elementary grasp of the principles and functional implications of AI agents and Agentic AI will be imperative to chart the future terrain of intelligent technology. The continuing development of these paradigms holds out the prospect of redefining our relationship with machines and our capacity to deal with complex issues, bringing with it an age of ever-more powerful autonomous intelligence.
Intelligent Automation with Heliosz.AI Agent Development Services
Are you ready to leverage smart automation to transform your business? Heliosz.AI offers full-spectrum AI agent development, plus maintenance, seamless integration, and strategic counsel. Our seasoned team develops original AI agents based on the latest LLMs and robust architecture, enhancing customer engagement, streamlining decision-making, and automating workflows. To ensure that your AI agents are intelligent, adaptive, secure, and fully embedded in your procedures, we provide complete support consisting of technical appraisals, tailored rapid engineering, effortless system integration, and ongoing optimization. Contact us today to speak about the possibilities and have Heliosz.AI assist you in developing the automation of the future.