The fusion of data analytics in marketing has given birth to a dynamic discipline known as data-driven marketing, and at the core of this transformation lies the profound influence of data analytics.
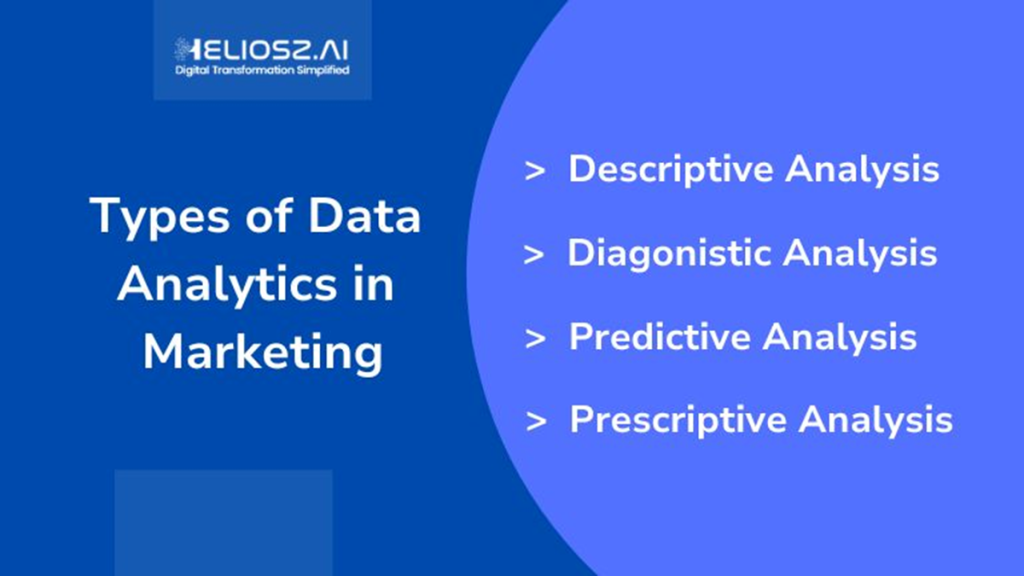
In this article, we delve into the world of data analytics and explore how it revolutionizes marketing strategies, backed by compelling statistics, and real-world examples.
- Harnessing Data Analytics for Modern Marketing
- Understanding Different Types of Data Analytics
- Real-world Examples of Data Analytics Success
- Unveiling the Power of Data in Marketing
- Overcoming the challenges on the path to success
Harnessing Data Analytics for Modern Marketing
In the digital landscape, data is generated at an unprecedented pace. Data analytics involves collecting, processing, and analyzing this data to uncover valuable insights.
These insights, when harnessed effectively, empower marketers to make informed decisions and tailor strategies that resonate with their target audience.
60% Increase in Conversion Rates with Data Analytics
Research indicates that companies implementing data analytics effectively have experienced an average 60% increase in their conversion rates. This underscores the potency of data-driven strategies in boosting a company’s bottom line.
87% of Marketers Believe Data Analytics is Essential
87% of marketers consider data analytics an indispensable tool for understanding customer behavior and preferences. The shift towards data-centric marketing is evident in the growing reliance on analytics-driven insights.
Understanding Different Types of Data Analytics
Descriptive Analytics
Reflects on past data to provide a retrospective view of marketing efforts.
Descriptive analytics serves as the foundation of data analysis in marketing. It involves examining historical data to gain a comprehensive understanding of past marketing efforts, campaigns, and customer interactions.
By scrutinizing this data, marketers can identify trends, patterns, and anomalies that shed light on what has happened in the past. This retrospective view is invaluable for assessing the effectiveness of previous strategies and making informed decisions moving forward.
For instance, a retail company might use descriptive analytics to analyze sales data from the holiday season to determine which products were the most popular among customers and which marketing channels yielded the highest conversion rates.
Diagnostic Analytics
Delves into the ‘why’ behind past performance by identifying patterns and correlations.
Diagnostic analytics takes the analysis a step further by focusing on uncovering the reasons behind certain outcomes and events.
It delves into the “why” questions, aiming to identify the root causes of past successes and failures. By examining patterns and correlations within the data, marketers can gain insights into what factors influenced specific outcomes.
Imagine an e-commerce platform experiencing a sudden drop in website traffic. Diagnostic analytics would help identify whether the drop was due to changes in search engine algorithms, seasonal fluctuations, or other external factors, enabling marketers to address the issue effectively.
Predictive Analytics
Employs past data to forecast future trends and outcomes.
Predictive analytics harnesses the power of historical data to forecast future trends, behaviors, and outcomes. By analyzing past patterns and extrapolating them into the future, marketers can anticipate customer preferences and behavior. This empowers them to make proactive decisions, allocate resources wisely, and tailor campaigns to align with upcoming trends.
For example, a mobile app developer might use predictive analytics to forecast user engagement patterns, helping them optimize updates and feature releases for maximum impact.
Prescriptive Analytics
Offers recommendations based on insights, guiding future marketing actions
Prescriptive analytics takes predictive analysis a step further by not only predicting future outcomes but also offering actionable recommendations to achieve desired results.
This type of analytics considers multiple variables and constraints to guide the best course of action. It assists marketers in making strategic decisions that are supported by data-driven insights.
Consider a social media marketing campaign that aims to increase brand awareness. Prescriptive analytics could recommend the optimal combination of content types, posting schedules, and engagement strategies based on historical data and market trends.
Real-world Examples of Data Analytics Success
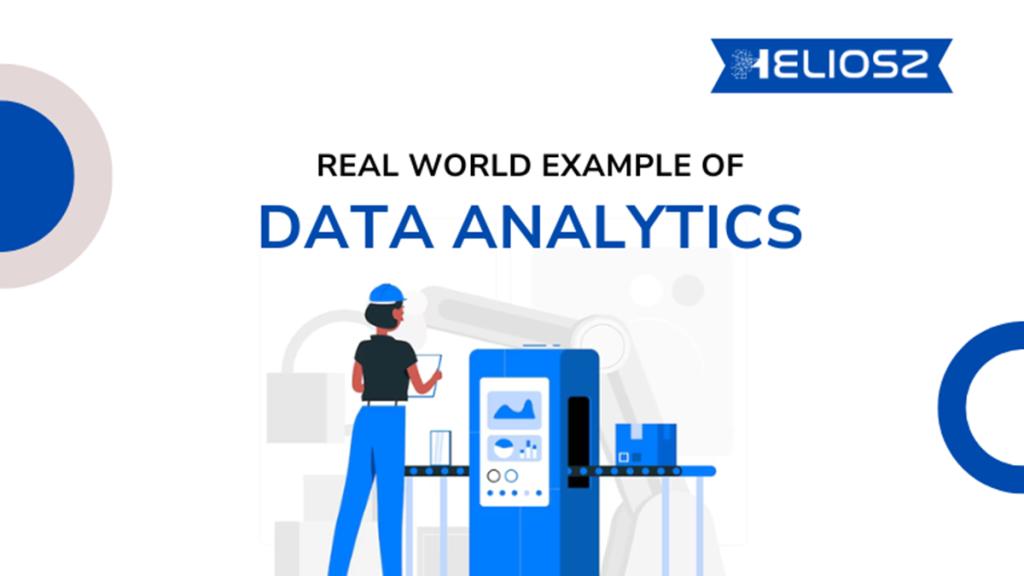
Amazon: The Master of Product Recommendations
Amazon’s recommendation system is a testament to the power of data analytics. By analyzing user behavior, purchase history, and even cursor movements, Amazon suggests products that customers are highly likely to buy, significantly boosting sales.
Netflix: Crafting Hyper-personalized Content
Netflix leverages data analytics to curate personalized content recommendations for its users. By analyzing viewing history, genre preferences, and even the time spent on specific shows, Netflix keeps viewers hooked and engaged.
Unveiling the Power of Data in Marketing
Personalization
Tailoring Experiences through Data
Data analytics enables marketers to craft personalized experiences for customers. By analyzing browsing history, purchase behavior, and demographic data, companies can deliver tailored recommendations and content, enhancing customer engagement.
Predictive Data Analytics in Marketing
Anticipating Customer Behavior
Predictive analytics leverages historical data to foresee future actions. Marketers can anticipate customer preferences and trends, optimizing campaign timings and product offerings accordingly.
Segmentation
Targeting the Right Audience
Through data segmentation, marketers can categorize their audience based on shared traits. This precision targeting ensures that marketing efforts are focused and relevant, leading to higher conversion rates.
Transforming Raw Data
Data storytelling is an emerging art. Marketers must not only gather insights but also present them in a way that captivates the audience, turning raw data into persuasive narratives that drive business growth.
Overcoming the challenges on the path to success
Data Quality and Accuracy
The effectiveness of data analytics hinges on the quality of the data collected. Inaccurate or incomplete data can lead to flawed insights and misguided marketing decisions.
Skill Gap and Training
There is a growing demand for skilled data analysts who can interpret and translate data into actionable strategies. Bridging this skill gap through training and education is a challenge that companies must address.
Conclusion
By deciphering intricate patterns and trends, businesses can create targeted strategies that resonate with their audience.
From personalization to predictive insights, data analytics is transforming marketing as we know it. As we move forward, embracing this data-driven evolution will be pivotal in staying ahead in the competitive landscape.
FAQ’s
What is data analytics in marketing?
Data analytics in marketing refers to the practice of collecting, processing, and analyzing data to gain insights that inform marketing strategies and decisions.
How does data analytics improve conversion rates?
Data analytics helps identify patterns and preferences among customers, enabling marketers to tailor their approaches, resulting in higher conversion rates.
What role does AI play in data analytics for marketing?
AI enhances data analytics by automating processes, identifying intricate patterns, and providing more accurate predictions for future marketing trends.
What are the challenges of data-driven marketing?
Challenges include ensuring data accuracy, navigating privacy concerns, and bridging the skill gap for proficient data analysis.
How does data storytelling impact marketing strategies?
Data storytelling transforms complex data insights into compelling narratives, making it easier for businesses to communicate their findings and drive strategic decisions.